AI in anti-money laundering efforts: a game changer
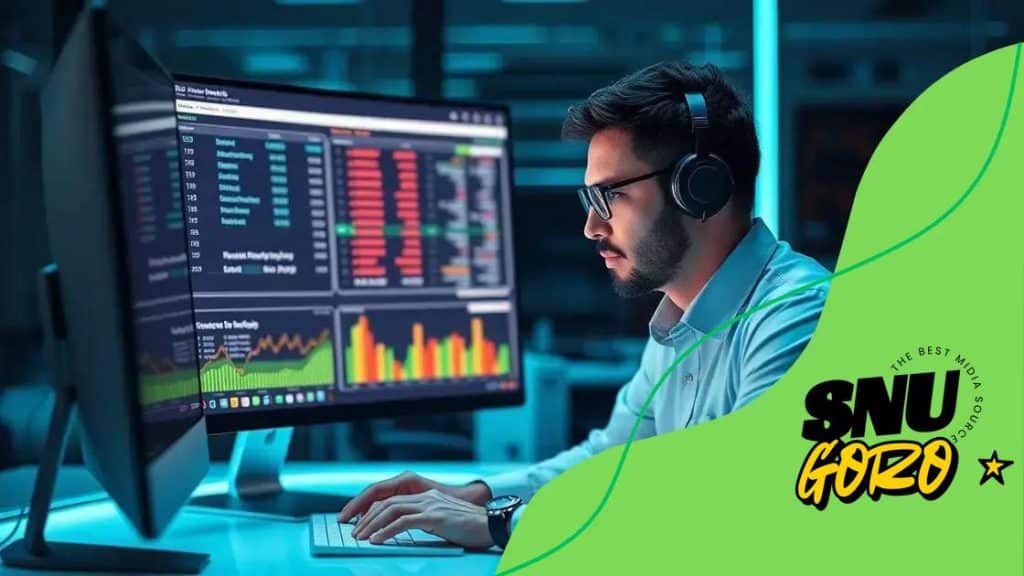
AI in anti-money laundering efforts enhances detection of financial crimes through advanced algorithms that analyze transaction patterns, enabling faster responses and improved compliance for financial institutions.
AI in anti-money laundering efforts is transforming the way financial institutions combat illicit activities. Isn’t it fascinating how technology can enhance security and vigilance? Let’s dive into this significant shift.
Understanding AI in the context of financial crimes
Understanding AI in the context of financial crimes can significantly improve detection methods. It plays a vital role in keeping financial systems secure.
As more businesses become targets for illicit activities, the need for effective solutions grows. AI technology is increasingly being used to combat these challenges.
What is AI’s Role?
AI analyzes large volumes of data quickly. By using advanced algorithms, it identifies unusual patterns that could signify suspicious behavior. This process is vital for staying ahead of criminals.
Benefits of AI in Financial Crime Prevention
- Improved accuracy in detecting fraud.
- Reduction of false positives.
- Faster response times to potential threats.
- Enhanced compliance with regulations.
With machine learning, algorithms continuously learn from new data. This ensures that detection capabilities keep improving over time. As AI refines its understanding, it can spot even the most elaborate schemes that humans might miss.
Furthermore, the integration of AI tools enables financial institutions to monitor transactions in real-time. This capability allows for immediate action, such as freezing accounts or alerting authorities when red flags are detected. Ultimately, this creates a safer environment for all users and maintains trust in financial systems.
The collaboration between technology experts and compliance teams is crucial. By combining human insights with AI’s power, institutions can create robust strategies that significantly hinder financial crimes.
How AI identifies suspicious transactions
How AI identifies suspicious transactions is crucial in the fight against financial crimes. By harnessing technology, financial institutions can efficiently detect potential fraud and money laundering activities.
Many transactions occur every second, making it challenging for human analysts to monitor everything. This is where AI shines, as it can process vast amounts of data much faster than a person.
Algorithms at Work
Machine learning algorithms are designed to learn from historical data. They study patterns of normal behavior and flag anything that deviates from these patterns. This helps in spotting anomalies that indicate suspicious activities.
Patterns and Anomalies
- Unusual transaction amounts.
- Sudden changes in transaction frequency.
- Transactions in high-risk locations.
- Multiple accounts linked to the same individual.
The combination of these factors can help uncover hidden threats. When AI identifies such anomalies, it triggers alerts for further investigation.
Additionally, AI systems continuously adapt and improve their detection capabilities. They analyze feedback from past alerts to refine their algorithms and reduce false positives. This means that over time, AI becomes more proficient at identifying genuine threats while minimizing unnecessary alerts.
The integration of AI technology ensures that financial institutions stay ahead of criminal intent. With advanced tools at their disposal, they can not only react faster but also preemptively thwart potential illegal activities.
Real-world examples of AI applications
Real-world examples of AI applications in combating financial crimes illustrate how effective this technology can be. Various organizations are leveraging AI to enhance their anti-money laundering efforts.
One notable example is a global bank that implemented an AI-driven system to analyze customer transactions. This system flagged suspicious patterns much faster than traditional methods. The bank reported a significant increase in the number of suspicious transactions identified, demonstrating the power of AI in real-time monitoring.
Successful Use Cases
Several industries have adopted AI technologies effectively. Some successful use cases include:
- Credit Card Fraud Detection: Several credit card companies use AI to monitor transaction data in real-time. By quickly analyzing spending habits, the system can detect unusual activity and prevent fraud.
- Insurance Claims Monitoring: Insurance companies use AI algorithms to identify fraudulent claims by examining patterns and anomalies.
- Trade Surveillance: Financial institutions employ AI to monitor trading activities and detect insider trading or market manipulation.
- Customer Behavior Analysis: Retailers use AI tools to analyze customer purchasing patterns, helping them identify potential money-laundering activities.
Additionally, a recent partnership between tech firms and regulatory bodies illustrates the commitment to using AI for compliance. These collaborations aim to create more robust systems that adapt to evolving threats.
As organizations share their successes, more industries realize the potential of AI technologies. This ongoing trend emphasizes the crucial role of artificial intelligence in building safer financial environments.
Challenges of integrating AI in compliance
Integrating AI in compliance presents several challenges that organizations must navigate. While AI technologies offer numerous benefits for detecting financial crimes, several hurdles can hinder effective implementation.
One significant challenge is the complexity of regulatory requirements. Financial institutions must ensure that any AI system adheres to laws and regulations, making compliance a central concern. As regulations evolve, adapting AI systems can be a daunting task.
Data Quality and Access
Another critical issue is the availability of high-quality data. Effective AI algorithms require large amounts of clean and accurate data to function properly. Organizations often struggle to collect, clean, and maintain this data. Without robust data, AI systems may produce unreliable results.
Technological Limitations
Additionally, existing technology might not be sufficient to fully leverage AI capabilities. Many organizations have legacy systems that are incompatible with modern AI** tools. This can lead to difficulties in integrating new technologies and limit their functionality.
- Resistance to change within organizations can further complicate adoption.
- Training staff to effectively use AI systems can require significant resources and time.
- Concerns about data privacy and security arise as sensitive information is processed by AI technologies.
Moreover, the potential for bias in AI algorithms raises ethical concerns. If training data contains biases, AI systems may inadvertently perpetuate these biases, resulting in unfair treatment of individuals.
Organizations must navigate these challenges while striving to harness the power of AI. Developing clear strategies for overcoming these obstacles is essential for successful integration.
Future trends in AI-driven anti-money laundering
Future trends in AI-driven anti-money laundering show a promising path for the financial industry. As technology advances, financial institutions are increasingly adopting AI solutions to detect and prevent illicit activities.
One key trend is the integration of predictive analytics. This approach allows banks to anticipate suspicious activities before they occur. By leveraging historical data and patterns, AI systems can provide early warnings, enabling proactive measures.
Enhanced Machine Learning Models
As machine learning evolves, models will become more sophisticated. They will better understand complex behaviors associated with money laundering. Improved algorithms will help minimize false positives, allowing compliance teams to focus on genuine threats.
Real-time Monitoring
Real-time monitoring will become more standard in the coming years. With AI technology, financial institutions can analyze transactions as they happen. This capability will allow for immediate responses to suspicious activities, improving overall compliance.
- Blockchain Analysis: Many firms are exploring ways to use AI with blockchain technology to enhance transparency.
- Collaboration Between Institutions: Sharing data among banks and regulatory bodies will improve overall security.
- Customer Behavior Insights: Using AI to analyze customer behavior can help differentiate between legitimate and illegal transactions.
Moreover, the increasing focus on data privacy will influence how AI applications are developed. Ensuring compliance with regulations like GDPR while utilizing customer data for analysis will be essential.
Overall, as technology continues to advance, the financial industry must adapt to these trends. Embracing AI-driven solutions in anti-money laundering efforts can lead to a more secure and compliant financial environment.
FAQ – Frequently Asked Questions about AI in Anti-Money Laundering
How does AI improve transaction monitoring in financial institutions?
AI enhances transaction monitoring by quickly analyzing large amounts of data, identifying suspicious patterns, and reducing false positives.
What challenges do organizations face when integrating AI into compliance processes?
Organizations face challenges such as regulatory compliance, data quality issues, and the need for employee training to effectively use AI systems.
How can AI help in detecting financial crimes more effectively?
AI helps detect financial crimes by using machine learning algorithms that continuously learn from patterns in transaction data, making detection more accurate and timely.
What are the future trends for AI in anti-money laundering?
Future trends include predictive analytics for early detection, real-time monitoring, and the integration of AI with blockchain technologies for enhanced security.