Advances in AI-powered healthcare diagnostics you should know
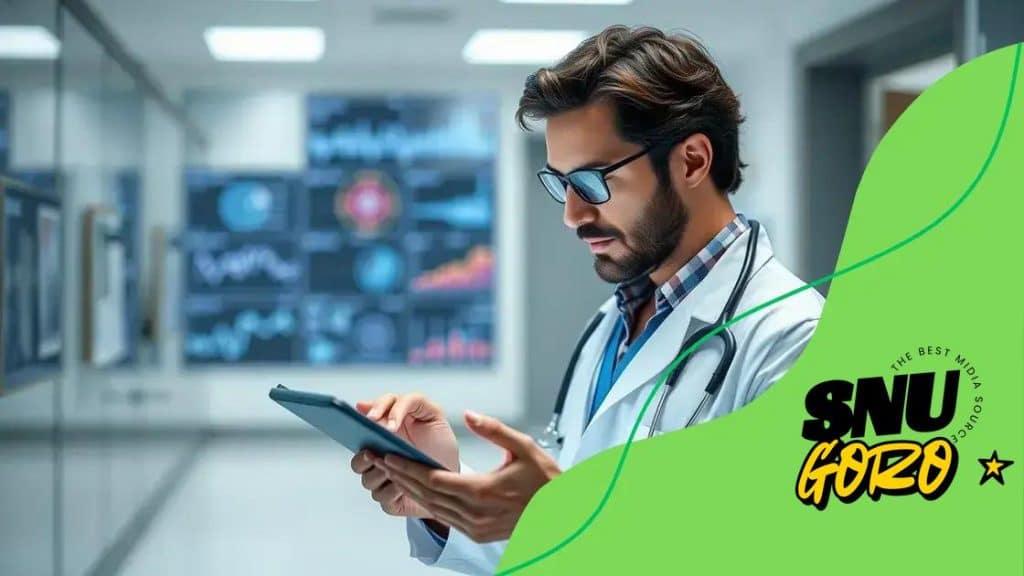
Advances in AI-powered healthcare diagnostics are revolutionizing patient care by enhancing diagnostic accuracy, personalizing treatment options, and streamlining healthcare processes while addressing challenges such as data quality and algorithm biases.
In recent years, advances in AI-powered healthcare diagnostics have made significant waves in the medical field. These innovations raise intriguing questions about how they can enhance patient care and improve outcomes. What could this mean for the future of healthcare?
How AI is revolutionizing diagnostic imaging
Artificial intelligence is changing the landscape of diagnostic imaging rapidly. With the ability to analyze vast amounts of data, AI is enhancing the accuracy and efficiency of medical imaging.
Improved Image Analysis
AI algorithms can identify patterns in images that may go unnoticed by the human eye. This leads to earlier detection of conditions like cancer. One major benefit is the speed at which AI analyzes images. Radiologists can focus on cases that require their expertise while AI handles routine evaluations.
Applications in Radiology
AI’s influence extends into several fields of radiology:
- Detection of tumors in mammograms.
- Classification of lung conditions in chest X-rays.
- Automated analysis of MRIs and CT scans.
- Enhancing image quality for clearer results.
As AI technology continues to develop, its ability to manage complex imaging tasks increases, leading to more accurate diagnoses.
Moreover, training AI systems requires vast datasets. Healthcare providers generate these datasets through daily imaging practices. Through shared learning, systems grow smarter and more reliable, assisting doctors in decision-making.
Enhanced Workflow Efficiency
The integration of AI into imaging workflows considerably enhances efficiency. With rapid analysis times and accurate results, healthcare professionals can streamline their processes. This means less waiting time for patients and more effective treatment pathways.
More importantly, AI provides valuable insights that assist in treatment decisions. For example, when radiologists receive AI-generated reports accompanied by imaging analysis, they can make informed choices faster. This synergy not only boosts confidence but improves overall patient outcomes.
Addressing Challenges
While AI is beneficial, challenges remain. Ensuring data security and addressing biases in algorithms are key issues. It requires ongoing collaboration between technologists and healthcare professionals to ensure AI tools are effective and fair.
In summary, AI’s role in diagnostic imaging is transformative. It offers precision and efficiency, making significant impacts on patient care and diagnosis.
The role of machine learning in medical diagnostics
Machine learning is becoming a vital part of medical diagnostics. By using algorithms and statistical models, it can assist healthcare professionals in making informed decisions quickly and accurately.
How Machine Learning Works
The essence of machine learning lies in its ability to learn from data. It analyzes previous cases and identifies patterns within them. This capability allows doctors to detect diseases early and improve treatment outcomes. Additionally, machine learning can handle large datasets, making it easier to extrapolate meaningful insights.
Applications in Diagnostics
Machine learning is being applied in various diagnostic fields:
- Identifying skin conditions through image recognition.
- Predicting heart disease based on patient history and symptoms.
- Analyzing lab results for quicker diagnoses.
- Monitoring chronic diseases with predictive analytics.
Moreover, the integration of machine learning into diagnostic tools empowers healthcare providers. As these systems grow smarter, they reduce human error and enhance the reliability of diagnostics. For instance, a machine can analyze thousands of X-rays in a fraction of the time it would take a radiologist.
The collaboration between human expertise and machine learning creates a powerful synergy. Physicians can focus on patient care while machines process complex data. The efficiency gained through this partnership is invaluable, allowing for more accurate diagnostics and timely treatments.
Challenges and Considerations
Despite its advantages, integrating machine learning into medical diagnostics comes with challenges. Data privacy is a significant concern, as patient information must remain secure. Additionally, biases in the algorithms can lead to unequal healthcare outcomes. Therefore, continuous monitoring and adjustments are essential to ensure equity in diagnostics.
The future of machine learning in medical diagnostics looks promising. As technology advances, we can expect even more revolutionary changes that will reshape how healthcare is delivered and experienced.
Examples of AI success stories in healthcare
Artificial intelligence has proven its value in healthcare through numerous success stories. These examples showcase how AI is enhancing patient outcomes and improving medical services.
AI in Diagnostics
One notable success story involves the use of AI in diagnosing diseases. For instance, a system developed for detecting diabetic retinopathy can analyze retinal images quickly and accurately. This early detection can help prevent blindness, highlighting the profound impact of AI on patient welfare.
Streamlined Administrative Processes
AI has also transformed administrative tasks in healthcare settings. Hospitals are using AI to improve scheduling and patient flow:
- Reducing wait times for patients.
- Optimizing the use of hospital resources.
- Automating billing processes.
- Enhancing patient communication through chatbots.
These innovations lead to better service, allowing healthcare professionals to focus more on patient care rather than paperwork.
AI in Personalized Medicine
Another exciting example is personalized medicine. AI analyzes genetic data to tailor treatments to individual patients. By understanding a patient’s unique profile, doctors can select the most effective therapies. This approach enhances treatment efficacy and minimizes side effects.
For example, AI algorithms can analyze cancer patients’ profiles to recommend targeted therapies, offering hope for more effective treatment options. The shift towards personalized care is a game-changer for many patients.
Efficient Drug Discovery
AI is making strides in drug discovery as well. Traditional methods can take years to bring a new drug to market. However, AI streamlines this process:
- Identifying potential drug candidates faster.
- Predicting interactions and effectiveness more accurately.
- Reducing costs associated with research and development.
These advancements are revolutionary, leading to faster and more cost-effective solutions to health challenges.
Through these examples, it is clear that AI is playing a pivotal role in reimagining healthcare. By improving diagnostics, streamlining processes, personalizing medicine, and transforming drug discovery, AI is not just a tool—it’s a partner in enhancing patient care.
Challenges faced by AI in diagnostics
Despite the benefits, the integration of AI in medical diagnostics faces several challenges. These hurdles can impact the effectiveness and reliability of AI technologies in healthcare.
Data Quality and Availability
One major challenge is the quality of data fed into AI systems. Poor quality or incomplete data can lead to inaccurate diagnoses. Healthcare organizations often struggle to compile comprehensive datasets because:
- Data may come from various sources with inconsistent formats.
- Patient privacy regulations can limit data sharing.
- Many healthcare providers still use paper records instead of digital systems.
Relying on flawed data can undermine the potential of AI and compromise patient safety.
Bias in Algorithms
Another significant issue is bias in AI algorithms. If the training data reflects existing biases in the healthcare system, the AI may perpetuate these biases. This can result in:
- Incorrect diagnoses for certain demographics.
- Inadequate treatment recommendations.
- Widening healthcare disparities among different patient groups.
Addressing bias is essential to ensure that AI tools provide equitable healthcare solutions for all patients.
Interpretability of AI Decisions
Healthcare professionals often find it difficult to understand how AI systems arrive at specific conclusions. This lack of interpretability poses risks because:
- Doctors may hesitate to trust AI-generated recommendations.
- Explaining AI decisions to patients can be challenging.
- Regulatory bodies require transparency before approving AI tools.
Enhancing the interpretability of AI decisions is crucial for building trust and ensuring effective use in clinical settings.
Integration with Existing Systems
Integrating AI into existing healthcare systems can be complex. Many facilities have legacy systems that do not easily accommodate new technologies. This leads to:
- Increased costs for upgrades and maintenance.
- Resistance from staff accustomed to traditional methods.
- Potential disruptions to patient care during the transition.
Successful integration requires collaborative efforts among technology providers, healthcare leaders, and staff to create seamless workflows.
Overcoming these challenges is essential for maximizing the potential of AI in diagnostics. By addressing data quality, bias, interpretability, and integration issues, the healthcare industry can enhance the effectiveness and reliability of AI tools, ultimately benefiting patient care.
Future trends in AI-powered healthcare diagnostics
The future of AI-powered healthcare diagnostics is bright and full of possibilities. As technology advances, several exciting trends are emerging that will shape the next generation of medical diagnostics.
Increased Personalization
One major trend is the move towards personalized medicine. AI will analyze vast amounts of data, including genetic information, to tailor treatment plans for individual patients. By understanding a person’s unique health profile, doctors can prescribe the most effective therapies. This approach leads to better outcomes and minimizes potential side effects.
Enhanced Predictive Analytics
AI is also expected to improve predictive analytics in healthcare. By utilizing machine learning algorithms, healthcare providers can better anticipate patient needs and potential health issues. This can result in:
- Early intervention strategies for at-risk patients.
- Improved resource allocation within healthcare facilities.
- Better management of chronic diseases through ongoing monitoring.
These advancements not only enhance patient care but also increase the efficiency of healthcare systems.
Integration with Wearable Technology
With the rise of wearable technology, AI will play a critical role in monitoring health in real-time. Devices like smartwatches and fitness trackers collect valuable health data that can be analyzed by AI systems. This integration will allow for:
- Continuous health monitoring outside of clinical settings.
- Immediate feedback for patients regarding their conditions.
- Proactive adjustments to treatment plans based on real-time data.
Wearable technology will empower patients to take control of their health and work closely with their medical teams.
AI-Driven Imaging Innovations
Future advancements in imaging technologies will likely enhance the role of AI. Innovations may include:
- Advanced imaging techniques that provide clearer and more detailed results.
- Automated analysis of imaging results, reducing the time required for evaluations.
- Real-time interpretation of images during medical procedures.
These innovations will significantly improve diagnostic accuracy and treatment planning.
As we look ahead, it is clear that the integration of AI into healthcare diagnostics will continue to evolve. The trends highlighted here promise a future where diagnostics are more accurate, personalized, and efficient, ultimately leading to improved patient outcomes.
FAQ – Frequently Asked Questions about AI-powered Healthcare Diagnostics
How is AI improving medical diagnostics?
AI enhances medical diagnostics by analyzing data faster and identifying patterns that may be missed by human eyes, leading to quicker and more accurate diagnoses.
What are the main challenges faced by AI in healthcare?
Key challenges include data quality, algorithm biases, the interpretability of AI decisions, and the integration of AI systems into existing healthcare practices.
Can AI personalize treatment options for patients?
Yes, AI can analyze individual patient data to recommend personalized treatment plans that improve outcomes and minimize side effects.
What future trends can we expect in AI healthcare diagnostics?
Future trends include increased personalization of medicine, enhanced predictive analytics, integration with wearable technology, and innovations in imaging techniques.